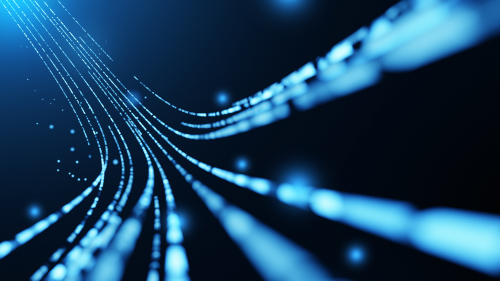
The Growing Importance of Metadata Management Systems
Introduction
As companies embrace digital technologies to transform their operations and products, many are using best-of-breed software, open source tools, and software as a service (SaaS) platforms to rapidly and efficiently integrate new technologies. This often means that data required for reports, analytics, and machine learning (ML) reside on disparate systems and platforms. As such, IT initiatives in companies increasingly involve tools and frameworks for data fusion and integration. Examples include tools for building data pipelines, data quality and data integration solutions, customer data platform (CDP), master data management, and data markets.
Collecting, unifying, preparing, and managing data from diverse sources and formats has become imperativein this era of rapid digital transformation. Organizations that invest in foundational data technologies are much more likely to build solid foundation applications, ranging from BI and analytics to machine learning and AI.
In recent years, several technology companies developed internal metadata management systems and shared the challenges that led them to focus on metadata (this list includes: Airbnb's Dataportal, Netflix's Metacat, Uber's Databook, LinkedIn's Datahub, Lyft's Amundsen, WeWork's Marquez, Spotify's Lexikon). These companies were facing fragmented data landscapes, while growing teams of analysts, data scientists, and engineers were needing to build data and machine learning products. The blog posts announcing these metadata management tools made it clear that these companies have come to rely on these metadata systems to power an array of data and machine learning services.
Beyond the need to unify and tame data from diverse systems, other reasons for the resurgence in interest in metadata technologies include:
- Regulations like SR-11, GDPR, and the California Privacy Rights Act (CPRA) require organizations to manage data privacy, access, and control efficiently and at scale.
- Debugging and root cause analysis are essential for machine learning and AI applications. The advent of new regulations raises the possibility of audits, making tools for data governance, model governance, and data lineage particularly critical.
- Data governance at scale requires a certain level of automation, especially when many different software systems and platforms are involved.
- Data discovery is particularly important for productivity reasons. Many users spend significant time finding and understanding the right data. A good data discovery product can help in this regard.
In this post, we examine emerging tools for managing metadata and data governance.A CxO or a VP of R&D might ask themselves why they need a metadata management system at all: are existing data governance and data catalog solutions not adequate? We argue that solutions built on top of metadata management systems result in data governance systems that are global in scope. Metadata management systems provide end-to-end data governance solutions that cover source systems, data warehouses, data management systems, and data pipelines that power enterprise applications. Advanced data protection techniques including masking, differential privacy, data synthesis can be integrated. The resulting data catalogs will be comprehensive, and changes will immediately be reflected in dependency mappings between data assets. As a result, users (analysts, data scientists, and engineers) will be able to search and discover trustworthy data that complies with internal and external regulations.
Metadata Management Architecture
Metadata systems typically have three building blocks:
The first layer, unified schema, is for collecting data into a unified platform. Metadata needs to be collected from all systems, including operational systems, analytics systems, and other software. This layer has three components:
- Extract, load, transform (ELT) - Depending on how ELT is implemented, data collection can be done using a push (changes and updates are sent automatically) or pull (metadata ELT periodically extracts changes or updates) mechanism.
- Refinement and storage - A data management system stores all the metadata data in a format that will be easy to retrieve.LinkedIn, for example, found that a "knowledge graph of metadata unleashed many services and applications."
- Access - APIs or domain-specific languages for extracting data from the metadata system are used to build the upper layers, Data Catalog and Governance.
In 2015, academic researchers began pointing out the potential applications of metadata management systems for data governance and other areas of data management. As we noted, several technology companies have built systems to begin realizing this vision. Recent posts by teams behind metadata management systems at LinkedIn and Lyft highlight the power of providing users with tools for discovering, accessing, and consuming trusted data.At LinkedIn, a metadata management system "powers numerous mission-critical use cases."
The second layer, Data Catalog, organizes data into an informative, searchable, and trusted inventory of all data assets. A Data Catalog has the following components:
- Data description - A detailed description, including summaries, of all data elements.
- Data lineage - Dataflow for the origin and evolution of data. In large organizations with multiple levels of data dependencies, for example, change management and communication with downstream users is a challenge that can be addressed with a knowledge graph.
- Data version control - A system responsible for tracking changes in datasets over time.
- Data usage - Tracking data usage and consumption by human users or by applications and systems. Data usage includes the ability to observe the actual flow of data in an organization. Data usage and consumption tracking can also help build cost-management solutions.
The final layer, Governance, manages the availability, integrity, and security of data in enterprise systems, based on internal data standards and policies that control data usage. Effective data governance ensures that data is consistent and trustworthy, and doesn't get misused. This layer has four components:
- Data discovery - A service that includes detecting sensitive data across all data platforms, saving time and limiting risk from manual errors.
- Data protection - A collection of techniques to reduce the unnecessary spread and exposure of sensitive data while simultaneously maintaining its usability.
- Data access management - A fine-grained access control on the cell level thatmaintains adherence to organizational policy and regulations.
- Data quality - The accuracy, completeness, consistency, and relevance of data. Data quality tools help assess quality and fix issues in data.
Snapshot of Companies
Below is a partial list of companies that have solutions in the three building block layers we described. In this graphic, a company or an open source project that appears in one of the layers may only address a subset of the components in that layer. Moreover, some companies span multiple layers, but for the sake of space and clarity, we opted not to place them in all the layers for which they potentially have solutions.
Summary
In this post, we describe a new set of metadata management systems and how they will impact data governance solutions, data catalogs, and other enterprise data systems. We close this post with the following observations about the future of metadata management solutions:
- A couple of startups already focus on metadata management, and we expect more companies to follow.
- We believe metadata management systems will be the foundation for many data management applications, as outlined in Figure 2 above.
- As AI and data applications increasingly rely on disparate data sources, data governance solutions must be global in scope—in other words, end-to-end data governance solutions that cover source systems, data warehouses, data management systems, and data pipelines
- It's important to emphasize that business value lies in global data governance, which can be best achieved through a unified schema.
Assaf Araki is an investment manager at Intel Capital. His contributions to this post are his personal opinion and do not represent the opinion of the Intel Corporation. Intel Capital is an investor in Immuta. #IamIntel
Ben Lorica is co-chair of the Ray Summit, chair of the NLP Summit, and principal at Gradient Flow.