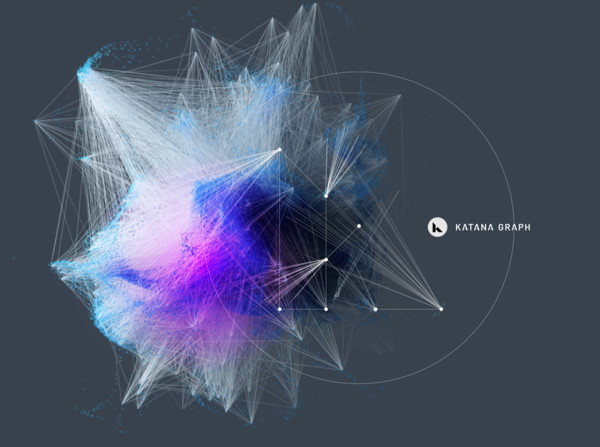
Contextualizing Data with Katana Graph
Graphs are a natural way to contextualize and explain data. Graph data is particularly beneficial when data is large, continually evolving, and rich with high-value relationships. However, for legacy reasons, most graph data is stored in relational databases without directly storing the relationship context and information, thus limiting data scientists' ability to seek deeper, smarter insights. Therefore, reliance on relational databases has limited enterprises from extracting timely insights from large data sets, which are unstructured and sparse. The amount of analytics and data mining that current systems provide is minimal, and they are intended to browse the data instead of getting insights by analyzing the data.
According to IDC1, by 2025, the world will generate 175 zettabytes of data, and the rate at which we are creating data is increasing explosively. As unstructured data continues to grow at 55%-65% CAGR, graph technologies' adoption is at an inflection point.
"Large, unstructured datasets are prevalent across a broad spectrum of use cases, from social network analysis, biomedical and pharmaceutical research, security and authentication, and many other applications," said Wei Li, Vice President and General Manager in the Architecture, Graphics, and Software group at Intel.
However, current approaches at gleaning insights from unstructured data sets have long been problematic. Traditional graph databases tried to address concerns with relational databases but, as referenced earlier, were not built with analytics in mind and were orders of magnitude slower than required. Enterprises quickly hit performance limits while traversing massive knowledge graphs or query processing even at low-latency and scale. Then along came the first wave of graph analytics startups focused on efficient data storage. Still, the primary bottleneck for adopting these technologies was a lack of performance and scale. Today, graph adoption is limited by a lack of tools, open standards, standardization around graph query languages, and the high cost of switching from legacy databases.
The graph ecosystem needs to evolve to close these technical gaps. We believe an accelerated graph computing platform is the key to obtaining timely insight from massive data sets. Superior performance for complex graph algorithms will require support for heterogeneous clusters of computing resources and ease of development of high-performance graph AI applications. To be successful, we have to build a scalable system and an end-to-end graph deployment platform with an integrated graph computing subsystem of a graph database, graph analytics, graph AI, and machine learning.
Katana Graph is a graph computing, AI, and analytics platform company, addressing the current graph technology challenges with an innovative, end-to-end approach in graph algorithms, storage technologies, hardware acceleration, and a sophisticated graph engine. The Katana Graph co-founders - Keshav Pingali (CEO) and Chris Rossbach (CTO) – are talented deep technology researchers and scientists with industry engineering experience. More than a decade of advanced research in graph technology and high-performance computing at the University of Texas at Austin has culminated in the technology behind Katana Graph. Keshav and Chris have built an incredible team, attracting talent from top technology companies and universities with graph algorithms, graph mining and AI, distributed computing systems, operating systems architects, and non-volatile memory file systems expertise.
We are excited about Keshav and Chris' vision, and we were thrilled to lead the group of investors in Katana Graph's Series A round.
We see an increasing enterprise demand for an end-to-end graph platform with a graph database, graph AI, graph analytics, and mining capabilities. Katana Graph is going after practical use cases in different verticals. Several enterprises are already utilizing the Katana Graph: intrusion detection, fraud detection, anti-money laundering applications – online security problems; implementing high-performance parallel modules to solve electronic circuit design problems; drug discoveries and genomics with 100B-1T edges; identity management with community detection applications (over 1 trillion edge graph).
Furthermore, Katana Graph announced its collaboration with Intel on enterprise-scale computing. "Our collaboration with Katana Graph to optimize its cutting edge technology on Intel processors including Xeon Scalable processors and GPUs will enable enterprises to gain deep insights at incredible speeds, at scale," said Wei Li.
We look forward to collaborating with the Katana Graph team as they transform the graph market with their competitive advantage in performance, scalability, programmability, and flexibility.