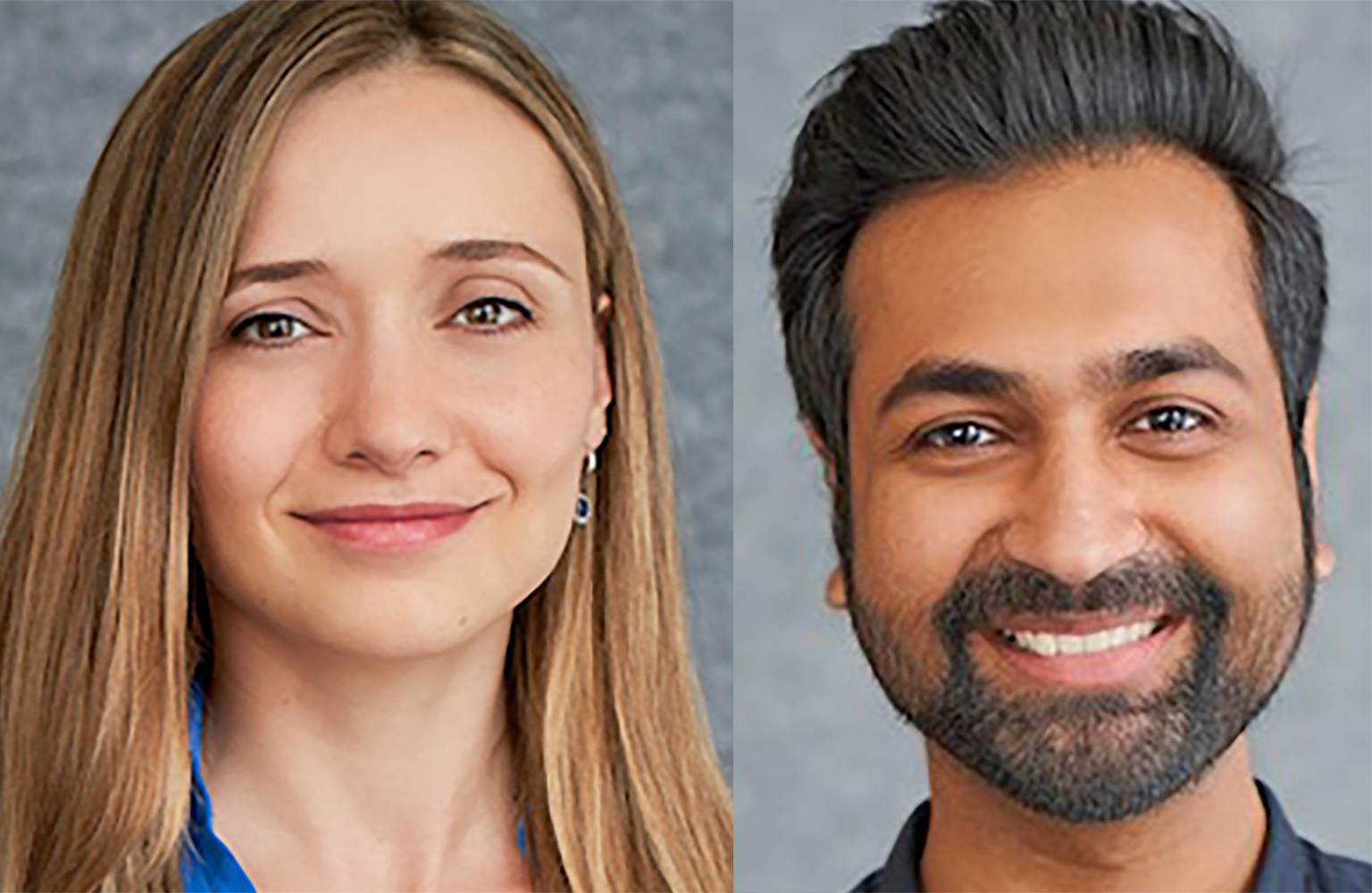
Same Same (APIs), but different (security level)
Productivity and scalability of confidential data
At Intel Capital, we are passionate about building a more efficient future. The ability to utilize data, create robust infrastructure and increase security will get us there – and Opaque hits the heart of all three. Fifteen months ago, we led the seed round for a multi-disciplinary founding team with tremendous experience securing data systems - and today, we’re proud to say that we have participated in their Series A.
Between security, flexibility, time limits, and data at odds, most organizations have to give up a few, and it's usually flexibility and timeliness. Opaque's platform creates a solution that removes these sacrifices and accelerates future technology. As a result, we see their platform greatly improving the efficiency of enterprises and companies like Intel and making a larger impact in the data analytics market.
The market demand
Confidential computing (CC) is a new and fast-growing market. According to a recent report by Everest Group, the confidential computing market was estimated at $2B in 2021 and is expected to grow at a CAGR of 90% to 95%. By 2026, the CC market is estimated to reach $54B. Such substantial market growth cannot occur only from a huge demand, but must rely on eliminating adoption barriers for the new technology. The database management system market is growing fast, with the open-source portion growing even faster.
Being a data-driven company means leveraging data assets and professional engineers to extract insights that will accelerate your growth. Due to privacy concerns and regulations, confidential data is typically locked on-premise and, therefore, inaccessible to users and to cloud tools. More than $300 billion of the world's most valuable data remains untapped due to the lack of a secure processing environment, creating a huge demand for solutions like Opaque.
Opaque’s eliminating the adoption barriers
For every new technology, the main barrier to adoption is the learning curve for the organization. If we could reduce the learning curve dramatically, we could accelerate the adoption. Born out of UC Berkeley's RISELab, Opaque Systems leverages a novel combination of two key technologies layered on top of state-of-the-art cloud security–secure hardware enclaves and cryptographic fortification. This combination aims to ensure that the overall computation is secure, fast and scalable.
Opaque's platforms enable commonly used data analytics APIs such as Spark SQL and XGBoost. Supporting existing APIs reduces the organization's learning curve in implementing new technology and keeps user and computation productivity high. Organizations can use Opaque to encrypt their confidential data on-premises, upload the encrypted data to the cloud, and then analyze the encrypted data on the cloud with their common tools and APIs. The platform's goal is to ensure that the data is never unencrypted during the lifecycle of the computation. Data owners retain full control over how their data is processed and full functionality to analyze data on their non-encrypted platforms.
Towards CC first-class citizen
Since its inception eighteen months ago, the Opaque team has focused on continuing to support the MC2 open source project (Multi-Party Collaboration and Coopetition). The open source software today is enterprise-ready with better usability as an MC² Client responsible for handling remote attestation and submitting jobs to the untrusted compute cluster and improved performance of the MC² Compute Services. On the product side, we have seen a growing inbound interest from enterprises with regulations on data movement and sharing from verticals like financial services and healthcare.
We remain committed to the fast growth pace of the CC market and welcome Walden Catalyst Partners, Storm Ventures, and Thomvest Ventures as new partners in the Opaque journey. We continue to work with the Opaque team, Race Capital, The House Fund, and FactoryHQ to make CC a first-class citizen in data analytics and machine learning.